Pricing Optimization for Retail
Rewritten by Conor Doherty, September 2023.
A persistent issue in modern retail is the presence of a competitor, somewhere, selling a practically identical product at an extraordinarily low price. Common responses are to adjust prices in line with experience (the intuitive pricing model) and the faithful application of econometric formulas (the naïve rationalist pricing model). Both are manual and flawed: the former, chiefly, lacks scalability; the latter is often too rigid. Naïvely aligning prices with competitors’ is also not a sustainable strategy. Overarchingly, given the often symbiotic relationship between pricing and demand, retail pricing strategies should be one step in an overall automated supply chain optimization.
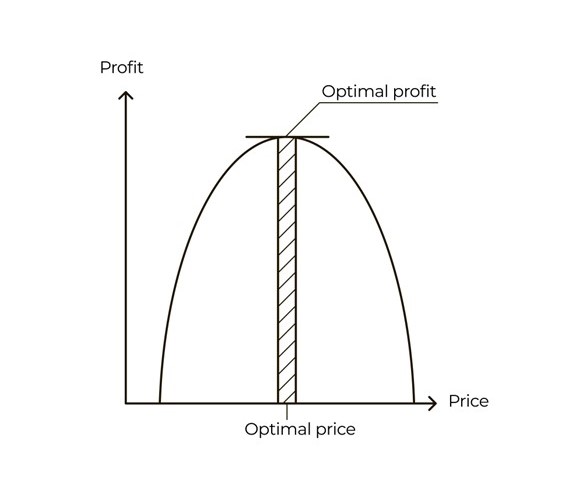
Retail Prices v Retail Pricing
Perhaps to the surprise of some, it is not retail prices but “pricing strategies” that can be optimized. The distinction between retail prices and retail pricing strategies is nuanced but critical, and frequently overlooked. Retail prices are contingent on multiple factors: purchase price, return rate, and competitor prices (amongst others), and these are highly fluid factors. Consequently, price Y could be an optimal price point for January but an inappropriate one for February. Given the ever-evolving market conditions, it might often seem impossible to conduct rigorous and effective pricing experiments.
A retail pricing strategy, however, is the logic that processes all relevant input data for a given business to compute the most applicable prices at that given moment. In reality, conducting rigorous pricing experiments is feasible, though it is not the prices that will be benchmarked, but the underlying pricing strategies.
For example, if one has 200 products and 2 retail pricing strategies, A and B, it is feasible to apply strategy A to half of the products and strategy B to the remainder. Prices fluctuate, possibly on a daily basis, according to strategies A and B. After a period of time, by comparing the respective results of A and B, it becomes feasible to determine which pricing strategy yielded superior results. This concept will be expanded upon in Quantitatively testing pricing strategies.
Two Pricing Antipatterns
In retail, pricing is often anchored to two approaches - the intuitive and naïve rationalist models. The former is the commonplace, gut-instinct methodology, while the latter is the textbook application of formulas and theorems. Each model may appear initially successful in response to pricing optimization quandaries, but ultimately beget equal - if not greater - problems as time progresses.
The Intuitive Pricing Model
The first errant approach - appealing more to hands-on-style practitioners - is the intuitive pricing model. Here, determining the appropriate price is a manual process that relies on the manager’s intuition (and likely several Excel spreadsheets as well). As this type of pricing strategy primarily exists in the mind of the manager, it is exceedingly challenging (if not outright impossible) to deploy this approach at scale, and the results are highly dependent on the manager’s judgement. Further, these intuitions - if successful - cannot be automated and, crucially, when the manager leaves the company, a considerable portion of the company’s valuable knowledge departs with them.
The Naive Rationalist Model
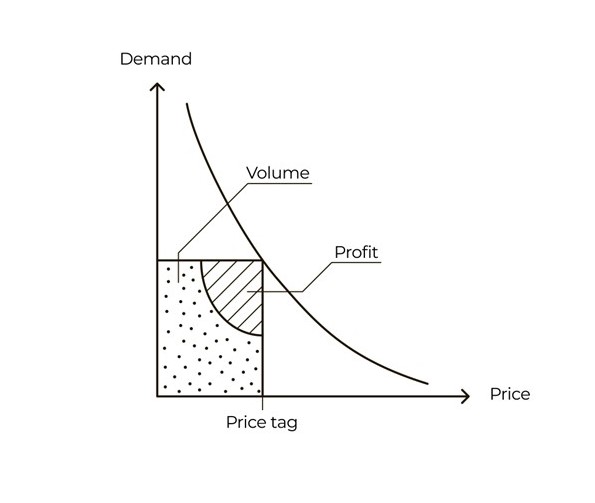
Figure 1: A textbook demand curve illustrating the balancing act between demand and price. As price (x-axis) increases, demand (y-axis) generally declines. When the market settles on an acceptable price, a certain level of volume (revenue) and profit can be expected.
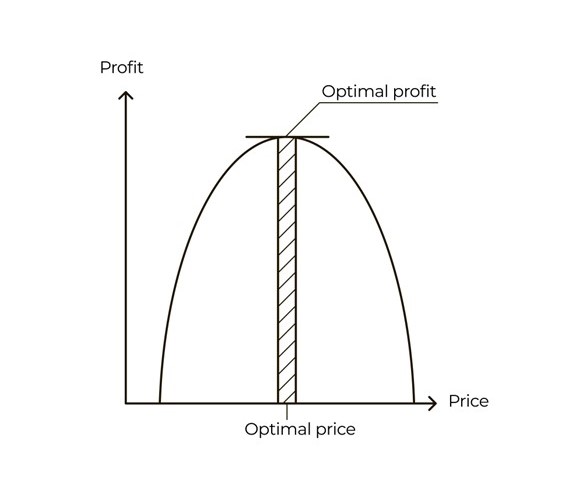
Figure 2: A curve conceptually depicting the optimal price for a product, with profit on the y-axis and price on the x-axis. This model (a theoretical extension of the market principles in Figure 1) purports not only to identify optimal profit points for a retailer but also - implicitly - demand . This curve attempts to treat prices as disembodied entities within the market and is a quintessential example of naïve rationalism in pricing.
Alternatively, one might be more inclined towards the academic perspective, in which case there is the naive rationalist pricing model. This approach deemphasizes instinct (such as the manager’s gut on a given day) in lieu of dispassionate, methodical econometrics. Much like the intuitive model described above, employing naïve rationalism in one’s pricing - such as that depicted in Figure 2 - presents entire classes of problems. Two key examples include:
-
Causality/Correlation: Establishing correlation between variables is relatively straightforward, but that does not automatically lead to the correct identification of causation. If one were to increase one’s prices in line with a given formula, there might be an instantaneous drop in sales. However, this might be unrelated to the rise in prices and attributable to the sudden emergence of a rival entering the market – something the formula did not (and possibly cannot) account for.
-
Simultaneity: Unlike the causality/correlation conundrum - where identifying the true cause of change is difficult when variables seemingly coincide - simultaneity refers to the problem of disentangling the first mover (used here in the Thomistic sense) when we already know two variables influence each other. For example, price and demand tend to influence each other, but a chicken-and-egg scenario can quickly emerge. When demand is high, one might increase prices, though this may subsequently reduce demand. This, in turn, might prompt one to lower prices only to see demand increase once again. This can result in an analytical regress that stymies pricing optimization.
Attempting to optimize pricing in this fashion can lead to an over-reliance on abstract, academic reasoning, where one employs a fresh formula with the emergence of exception or quandary and discounts the role of intangibles (such as sentiment). All this is to miss the root problem: pricing is a highly domain-specific matter and the intricate business contexts (including both quantitative and qualitative factors) must be incorporated into one’s pricing strategy to yield sensible results. The naive application of econometric formulas merely propels one in a different - and equally incorrect – direction1.
The Price Alignment Problem
An additional and enduring concern - one inadequately addressed by either model described in the previous section - is the threat of (most likely algorithmic) war presented by price alignment in competitive markets. This is even worse for retailers who do not own the brands they sell and are potentially one of several businesses offering identical products in the same region. Lowering prices to undercut one’s competitors will, in all likelihood, be nullified almost immediately by the competitors’ response: lowering their prices even further.
The implications of the price alignment problem in the present context are significant. Consider the following scenarios:
-
Intuitive model: An experienced manager using their intuition might have a solid understanding of their customer base, costs, and the historical performance of their products. However, if competitors frequently change their prices, and the manager has to adjust their own prices in response, this disrupts the manager’s established pricing strategy. The nuanced understanding the manager may have of the market is rendered moot by the blunt financial pressure to match (or undercut) the new market price. This constant price alignment can lead to lost sales or reduced profit margins.
-
Naive rationalist model: An experienced manager relying on textbook formulas might set prices based purely on formulas factoring costs and desirable profit margins, offering a seemingly rational approach to pricing. However, in a dynamic competitive market where identical products are sold and prices are regularly lowered, this naive rationalist model quickly defaults in much the same way as the intuitive one. Obdurately persisting with prices determined by optimal cost curves (for example), despite significant price shifts by competitors, will invariably lead to a loss of market share. Conversely, blind adherence to price alignment without considering customer price sensitivity (and other qualitative behaviors) could result in reduced sales.
In short, both intuitive and naïve rationalist approaches present significant limitations when the manager’s gut and theorist’s textbook face the complex interplay of large-scale, competitive market forces. This is to say nothing of the impact the price alignment problem can have on demand forecasting. In short, frequently updating one’s prices in a retail race to the bottom serves to exacerbate demand forecasting difficulties as greater uncertainty is introduced to demand forecasting models.
This, in fact, reveals the central misconception underscoring the pricing models described here: attempting to optimize prices in isolation - i.e., independent of initially optimized demand forecasting - is a somewhat backwards proposition. Whichever model one employs, the price alignment problem stands astride it.
Optimizing prices without also optimizing demand forecasting may very well lead to adherents of both models setting prices too high and suppressing demand (and thus profits), or setting prices too low and sacrificing profits. Therefore, establishing an effective, competitive pricing (and business) strategy must incorporate both demand and pricing optimization2.
Establishing a Pricing Strategy
Effectively optimizing one’s pricing strategy requires cogent defining and price testing. The former entails delineating precisely what one’s offering is within the broader market, as pricing strategies differ depending on the type of good/service one provides; regarding price testing , rigorous quantitative testing - factoring the multitude of salient variables governing consumer behavior - is imperative.
Defining the Product to be Priced
Underpinning one’s pricing strategy is the correct designation of the product itself. Goods can generally be divided between wants - things people desire to consume - and needs - things people generally must consume.
Markets of wants are typically characterized by sellers whose products are desired for purposes beyond mere function, and these products are closely tied to a brand. This brand is, in effect, the engine of demand. For example, a high-end bag from an exclusive designer can easily have its functionality replicated by a plastic bag from a local supermarket. However, this is to ignore the value people ascribe to the designer’s creation. Fundamentally, should a consumer opt not to purchase a product of want, there will be no adverse effects given the inherently discretionary nature of the purchase.
Markets of needs, conversely, essentially mandate consumption. Opting not to purchase electricity for one’s home is a choice with immediate - and potentially lasting - consequences. Consumers might (if their country’s energy market is liberalized) have options for their energy providers, but the need for consumption is practically guaranteed. In other words, as electricity is sold in a market of needs, there is going to be a provider that satisfies this demand. In these situations, brands (or companies) are engines of choice rather than demand.
Defining which market one operates in is a vital first step in calibrating one’s pricing strategy. Even after this is determined, one’s strategy still requires nuance. Consider the challenges strictly intuitive or naive rationalist pricing models face with luxury and essential goods.
For luxury items (wants), the intuitive model may overlook psychological value, while the naive rationalist model might undervalue consumer perception. For essential goods (needs), the intuitive model may lead to unfair pricing due to demand inelasticity3, while the naive rationalist model might not account for cost fluctuations or social impact of high/low prices.
Given pricing strategies will shift depending on wants versus needs (amongst a litany of other factors), the following sections will provide guidance applicable in most scenarios, though the fine print will naturally vary depending on particular clients’ situations.
Quantitively Testing Pricing Strategies
In a retail market of wants, A/B price testing can be used to identify an optimal price point that maximizes profit or sales. This process involves selecting a product (or products) and two different price points, then splitting the customer base into two similar groups, running the test (offering the product at each price point), and analyzing the results. The outcomes should consider quantitative metrics such as sales, revenue, and profit, as well as qualitative impacts like brand perception and customer satisfaction. For luxury goods (wants), a higher price point may drive fewer sales but might enhance perceptions of exclusivity and quality, leading to higher total profit.
In contrast, A/B price testing in a market of needs involves similar steps but requires extra care due to the inelastic demand of these products/services. Service providers can use this strategy to understand how changes in price affect customer behavior, satisfaction, and overall revenue. Yet, tests in this market must be conducted with ethical considerations, and the potential impact on customers’ ability to access essential goods or services (needs), in mind. Regulatory frameworks may also significantly influence such tests, and price adjustments should avoid causing undue hardship to customers.
Given that A/B tests are labor-intensive and costly, the focus should be on the incremental discovery of a pricing knowledge base, rather than exhaustive testing. This provides the opportunity to comprehend market responses to prices, with each experiment testing a single hypothesis and systematically yielding domain-specific conclusions. By amalgamating these insights, it becomes feasible to devise a comprehensive pricing strategy predicated on a deep, quantitative understanding of the market, as opposed to relying on an opaque numerical optimization toolkit photocopied from a university coursebook.
In practical terms, a pricing strategy consists of a series of simple insights, including:
-
Refraining from exerting pressure on prices when stock is dwindling
-
Halting AdWords expenditure on products that are not competitive for initial contact
-
Mitigating the accumulation of obsolete inventory by offering timely discounts
-
Ceasing promotions for products that could have been sold at full price
By judiciously applying these insights, retailers can create comprehensive pricing strategies, surpassing the limitations of intuitive or naive rationalist models.
Programmability is Inescapable
Considering market conditions are in constant flux, retail prices should reflect this dynamism. Any strategy that depends on manually adjusting individual prices suffers not only from the inability to be tested and consequently measured, but also from markedly low productivity. Therefore, the execution of pricing strategies should be predominantly automated.
Any software that automates retail pricing strategies must be equipped to accommodate virtually any conceivable strategy. If not, a business is consigned to a limited selection of pricing recipes that may not be ideally suited to its needs. To ascertain whether a given software is sufficiently powerful for specific requirements, a straightforward experiment – the Excel test – can be employed. According to this test, a pricing solution should be capable of implementing any pricing strategy that could otherwise be realized in Excel.
Succinctly, if a piece of software is incapable of executing tasks readily achievable in Excel, it is unreasonable to expect any sophisticated retail pricing capabilities arising from said software4.
Relevant Data for Pricing
A common tendency among most retail pricing tools is to accord significant importance to the prices set by competitors. Though this can be a valid source of information (which will be addressed in a later subsection), there is a danger of overweighting this data and ignoring the much larger picture. Plainly stated, the prices set by one’s competition do not necessarily provide lucid guidance on whether or not to match or undercut said competition. More importantly, they fail to indicate which products are crucial for executing such strategies, or the appropriate time and place for the implementation of a fresh pricing strategy.
Historical business data
Rather than simply anchoring prices to those of one’s competitors, a nuanced retail pricing strategy begins with an in-depth analysis of one’s own business data. This data is the most reliable and comprehensive source of information for any business, and a thorough accounting of one’s vast array of data streams can yield invaluable insights. In decreasing order of importance, these streams include:
-
A complete product catalogue (and their attributes)
-
Inventory stock levels and incoming purchase orders
-
Record of past items sold
-
Record of past purchase orders
-
Record of past cancellations, returns, chargebacks, and incidents
-
Record of past displayed prices
-
Web traffic aggregated per product per day
-
Search Engine Marketing (SEM) costs per product per day
Among this data, the latter two streams are typically more challenging to consolidate, primarily due to the sheer volume of relevant data, which is typically 100 times larger than all other datasets combined. However, except for these last two points, retrieving the aforementioned core business data should take absolute precedence over acquiring competitors’ data5.
Competitive Intelligence
Upon the acquisition and strategic deployment of core business data for the retail pricing optimization, a business can pivot to the meticulous surveillance of their competitors’ price action. From a technological standpoint, competitor price monitoring encompasses three distinct steps:
-
Crawling: This refers to the automated exploration of all accessible links on a competitor’s website, with the intent of uncovering every product offered to customers.
-
Feature Extraction: This operation entails the automated transformation of unstructured web pages into a structured dataset. It primarily focuses on isolating product names, product attributes, and product prices, among other salient elements.
-
Product Matching: This task involves the automated pairing of comparable products between a business’s offerings and those of their competitor(s). Of the three steps above, product matching often presents the greatest operational complexity, particularly for sectors where there are no direct product matches, such as in the realm of fashion6.
Given the availability of a robust IT team, it is feasible for a company to implement their own solution, utilizing open-source tools such as Scrapy.org. This resource provides a substantial advantage in terms of achieving tangible results within a short time frame7.
Limits of Competitive Intelligence
Pricing strategies predicated on core business data are desirable, given the quality of the underlying data is generally impeccable. Occasional inaccuracies may arise due to data entry errors but, on the whole, data can be considered absolutely accurate (essentially 100%).
Conversely, the quality of competitor data - even under optimal circumstances - tends to fall considerably short. Each step detailed in the previous subsection carries the potential for costly failure: a specific competitor product might be overlooked, an incorrect price may be extracted, or a product may be incorrectly matched. Considerable effort must be dedicated to the accurate appraisal of this data, otherwise the retail pricing optimization is at risk of significant data integrity issues.
Numerous vendors purport to offer pre-eminent competitive intelligence solutions. As a general guideline, conducting a straightforward test to challenge such claims is advisable: request the competitive intelligence vendor to provide a side-by-side comparison of one’s online store with that of one’s primary rival. This exercise allows for the assessment of the competitive intelligence technology by comparing freshly scraped data from the web with the data extracted from one’s own internal systems8.
Another indication of potentially weak technology is a vendor requesting an extract of a prospective client’s product database. In essence, the vendor likely seeks this information to ensure that the displayed data at the end of the pipeline aligns with the client’s product database. This practice is not only underhanded but also thwarts any attempts client-side to identify substantial data quality issues. In truth, the vendor is well aware that a given client’s ability to examine competitor pricing data is markedly limited, unlike the data from one’s own website that can be directly cross-referenced with internal databases.
Lokad’s Take
Attempting to discover optimal price points without first optimizing demand forecasting leaves one vulnerable to otherwise predictable problems, such as erratic customer behavior (e.g., seasonality) and brittle inventory management policies. As such, retail pricing should not be treated as an independent mechanism, but rather as part of an overall supply chain optimization. Within this framework, it is best to avoid pricing practices that do not scale (such as instinctive price-setting), and ones that are overly rigid and overlook the role of key qualitative forces.
Beyond this, developing an effective retail pricing strategy requires extensive and exacting analysis of one’s historical business data, careful price experimentation, and an automated approach to manage constant market changes. Moreover, data integrity, especially when it comes to competitor data, is paramount to avoid retail pricing missteps. For this reason, looking inward first - to one’s own business data - provides the best foundation upon which to erect a competitive retail pricing optimization strategy.
Lokad is engineered to optimize the entire supply chain, from prioritized inventory replenishment to retail stock allocation and competitive item pricing.
Supply chain practitioners looking to optimize their entire supply chain are welcome to email contact@lokad.com to arrange a demonstration of an end-to-end automated optimization.
Notes
-
Also known as scientism, this is an excessively sanguine belief in scientific (or scientific appearing) ideas. Abstract economic reasoning is helpful in the classroom, but the transition from academic vacuum to the aggressive theatre of economics is often as chasmic as it is disastrous. Pricing Optimization for the Automotive Aftermarket provides a detailed critique of the disconnect between econometric theory and praxis. It also demonstrates the coding involved in Lokad’s automated competitive price alignment approach. ↩︎
-
Demand forecasting optimization is beyond the scope of the present document, but it is worth planting a flag here regardless. See prioritized inventory replenishment to learn more about decision optimization with probabilistic forecasts. ↩︎
-
Demand inelasticity refers to a market situation where changes in price have little to no effect on the quantity of a good or service demanded by consumers. It typically applies to essential goods and services. Electricity is a prime example of demand inelasticity. ↩︎
-
Anecdotally, when pricing optimization software lacks programmability, managers (somewhat understandably) often simply revert to Excel. Lokad, for this reason, uses a compact programming language named Envision, which is specifically designed to tackle all facets of supply chain optimization, including pricing optimization strategies. ↩︎
-
On this note, Lokad extends native support for numerous enterprise resource planning applications. If a client’s preferred application is already supported, the bulk of historical data can be imported into Lokad with relative ease. If the client’s application lacks support, Lokad supports the uploading of tabular data files such as Excel spreadsheets or flat text files (e.g., .csv files ). Each Lokad account is equipped with a file-hosting service, enabling file import through web uploads or alternative protocols like FTP or SFTP. ↩︎
-
Consider the relative ease of comparing computers - in terms of price, functionality, weight, size, etc. - versus trying to methodically (and reliably) compare the relative merits of dresses. ↩︎
-
Lokad does not provide competitive intelligence services, though it can, naturally, factor a competitor’s data into a pricing strategy if the information is made available. If a client wishes to leverage the prices of rivals, the client needs to source and provide Lokad with this data. In practice, most clients obtain this data from one of the many competitive intelligence solutions that can be found on the Internet. Nearly all such solutions provide flat text file exports (e.g.,
.csv
files) of the pricing data, which perfectly align with Lokad’s data processing capabilities. ↩︎ -
One clear indication of vendors with subpar competitive monitoring technology is the absence of a free trial. The lack of such an offering implies a significant deficiency in automation. In fact, Lokad has witnessed many times over that merchants are better served by in-house web scraping solutions as opposed to opting for weak technology vendors. ↩︎